Data Science in Manufacturing (製造數據科學) / Intelligent Manufacturing
Systems (智慧型製造系統)
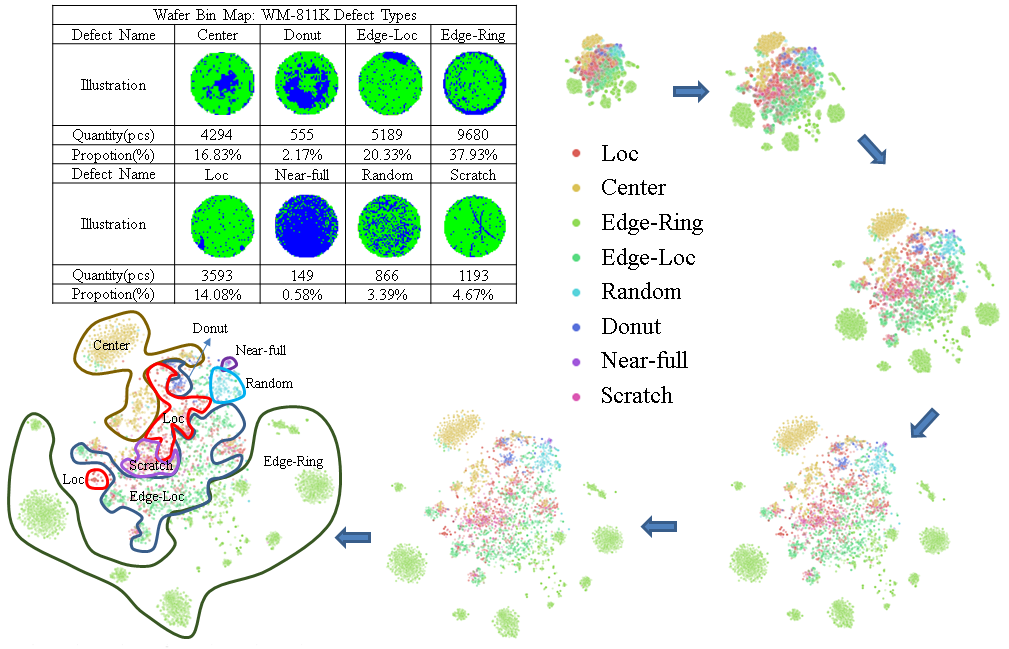
Manufacturing
Data Science (MDS) is a decision-oriented system which has the
computational
intelligence and self-learning ability to optimize the manufacturing
process. The
techniques include data science (decision tree, deep learning, feature
engineering, clustering, etc.), meta-heuristic algorithms (e.g. tabu
search,
simulated annealing, genetic algorithm, particle swarm optimization,
etc.),
fault detection & classification (FDC), statistical process control
(SPC), engineering data analysis (EDA), etc. These
methodologies can optimize resource allocation and support
trouble-shooting process. The applications are diversified such as
capacity planning, production scheduling, machine configuration
optimization, process fault diagnosis, facility layout, bottleneck
identification, quality and yield enhancement, market
segmentation,
pattern recognition, etc. Real-setting empirical studies were conducted
to validate the proposed model and improve the business core competence
effectively.
Research Interests:
- Data Science in Manufacturing (製造數據科學)
- Data Mining for Yield Improvement (數據探勘與良率改善)
- Statistical Process Control (SPC) Big Data Analytics (統計製程管制大數據分析)
- Process Diagnosis & Pattern Recognition (製程診斷與樣型識別)
- Price Forecasting and Optimal Decision by Reinforcement Learning (價格預測與強化學習)
- Virtual Material Quality Investigation (虛擬物料品質檢測)
- MECE Engineering Feature Selection and Predictive Maintenance (工程參數篩選與預測保養)
- Manufacturing System Management (製造系統管理)
- Multi-Objective Job-Shop Stochastic Scheduling (多目標隨機生產排程)
- Demand Forecasting and Robust Capacity Planning (需求預測與穩健產能規劃)
- Work Study and Time Motion Study (工作研究與時間動作研究)
- Vendor Selection and Order Allocation in Supply Chain (供應鏈廠商評選與訂單配置)
Representative Publications:
- Shen, Po-Cheng, Lu, Meng-Xiu, and Lee, Chia-Yen*, 2022. Spatio-temporal anomaly detection for substrate strip bin map in semiconductor assembly process. IEEE Robotics and Automation Letters, 7(4), 9493-9500.
- Lee, Chia-Yen, Chou, Bai-Jian,
and Huang, C.-F. 2022. Data science and reinforcement learning for price forecasting and raw material procurement in petrochemical industry. Advanced Engineering Informatics, 51, 101443.
- Lee, Chia-Yen, Wu, Chao-Shian, and Hung, Yu-Hsin, 2021. In-line predictive monitoring framework. IEEE Transactions on Automation Science and Engineering, 18 (4), 1668-1678.
- Hung, Shao-Yen, Chia-Yen Lee, and Yung-Lun Lin, 2020. Data Science for Delamination Prognosis and Online Batch Learning in Semiconductor Assembly Process. IEEE Transactions on Components, Packaging and Manufacturing Technology, 10 (2), 314-324.
- Lee, Chia-Yen and Bo-Syun Chen, 2018. Mutually-Exclusive-and-Collectively-Exhaustive Feature Selection Scheme. Applied Soft Computing, 68, 961-971.
- Lee, Chia-Yen
and C.-F. Chien, 2014. Stochastic Programming for Vendor Portfolio Selection and Order Allocation under Delivery Uncertainty. OR Spectrum, 36 (3), 761-797.
|
|

Productivity and Efficiency Analysis (生產力與效率分析)/ Production Economics (生產經濟學)
|
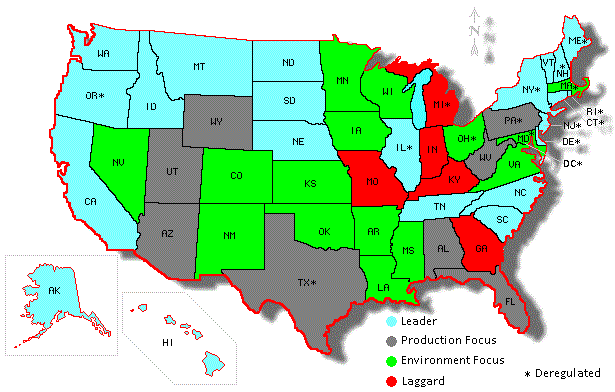 |
Productivity
and
efficiency analysis (PEA) is a discipline to assess
the performance of production system and drive productivity. The
technique is developed to estimate production function based on
production economics and then the efficiency of production system can
be identified. PEA supports evaluating the productive efficiency,
productive
effectiveness, product mix,
scale size, productivity change, performance benchmarking, market
power, etc. The research focuses
on developing nonparametric method (e.g. Data Envelopment Analysis,
DEA) and
semi-parametric method (e.g. Stochastic semi-nonparametric envelopment
of data,
StoNED) applied to the areas of manufacturing, airlines, energy market,
power system, cap-and-trade, carbon emission, biofuel diesel, etc.
Research Interests:
- Price for CO2? 一噸二氧化碳賣多少錢?
- Data Envelopment Analysis (數據包絡分析)/ Performance Evaluation (績效評估)
- Stochastic semi-nonparametric envelopment of data
(StoNED)
- Marginal Abatement Cost and Allocation of Emission Permit (邊際減排成本與排放權配置)
- Productive Efficiency, Effectiveness and Scale (生產效率、有效生產與最適規模)
- Marginal Profit/Productivity Analysis (邊際利潤/邊際生產力分析)
- Nash Equilibrium in Oligopolistic Energy Market (能源寡占市場下之納許均衡)
Representative Publications:
- Tseng, Chin-Yi, Lee, Chia-Yen*,
Wang, Qunwei, Wu, Changsong, 2022. Data envelopment analysis and stochastic equilibrium analysis for market power investigation in a bi-level Market. Transportation Research Part E: Logistics and Transportation Review, 161, 102705.
- Lee, Chia-Yen,
Ke Wang, 2019. Nash Marginal Abatement Cost Estimation of Air Pollutant
Emissions Using the Stochastic Semi-Nonparametric Frontier. European Journal of Operational Research, 273 (1), 390-400.
- Lee, Chia-Yen. 2019. Decentralized Allocation of Emission Permits by
Nash Data Envelopment Analysis in the Coal-Fired Power Market. Journal of Environmental Management, 241, 353-362.
- Lee, Chia-Yen and Peng Zhou, 2015. Directional Shadow Price Estimation of CO2, SO2 and NOx in the United States Coal Power Industry 1990-2010. Energy Economics, 51, 493-502.
- Lee, Chia-Yen, 2015. Distinguishing Operational Performance in Power Production: A New Measure of Effectiveness by DEA. IEEE Transactions on Power Systems, 30 (6), 3160-3167.
- Lee, Chia-Yen, 2014. Meta-Data Envelopment Analysis: Finding a Direction Towards Marginal Profit Maximization. European
Journal of Operational Research, 237 (1), 207-216.
|

|